Introduction
In the emerging era of big data, vast amounts of data are available in many kinds of databases. Unfortunately, many users who have access to this data are unable to use it effectively because they do not know how to extract relevant, concise and comprehensible features or summaries of the data; that is, they do not know what queries to formulate in order to discover novel and useful aspects of the data. This issue can be addressed in part by a system that takes positive and negative example tupleswhich is generally easy for users to provideand returns concise, comprehensible SQL queries that classify the provided tuples in simple and potentially interesting ways.
The creation of queries from examples can be thought of as adata miningclassification problem, which is often one task within a larger knowledge discovery in databases process (Freitas, ). In this task the objective is to create a comprehensible and interesting query that correctly classifies the given examples. In many cases we have no reason to expect there to be a simple query that perfectly classifies the examples, but we would nonetheless like to create a reasonably simple query that both does a good job at classifying the examples and is concise enough to be easily interpreted by the user.
To make the general problem more concrete, we seek a system that takes as inputs a database D and training example tuples

where E D and
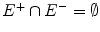
. Here, E + is the set of positive examples, and E is the set of negative examples. The goal of the system is to discover a concise and potentially interesting query Q such that E + Q ( D ) and
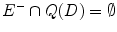
.
We have developed a system called Query From Examples (QFE) that takes the set of examples E and searches for a query Q that satisfies the above properties. It does this by means ofdevelopmental genetic programming. In QFE, each program P creates (or develops) a query Q P that is then evaluated on how well it correctly classifies the given example tuples E .
In contrast to other approaches to the production of database queries with GP (see below), this form of developmental GP allows QFE to use standard program representations and genetic operators, along with standard population and evolutionary control parameters. The only change required to use this approach in conjunction with most GP systems is to include new developmental functions in the systems function set. The developmental approach makes it easy to implement systems like QFE on top of existing GP systems and thereby to take advantage of advances in the general state of the art of GP. In addition, it may make it easier to evolve queries of arbitrary structure, thereby enhancing the generality of the system for a wide range of applications.
In the work described in this chapter we ran QFE on a standarddata miningclassification task and compared its results to those given by thedecision tree classifier C5.0. We find that although QFE does not produce quite as accurate a classifier as C5.0, the classifier that it produces is more concise and comprehensible than the one produced by C5.0. We therefore believe that developmental GP is competitive with, and in some ways superior to, other modern data mining systems on the creation of classifiers.
The remainder of the chapter is structured as follows. The next section describes work that others have done evolving SQL queries. Section Evolving Queries from Examples describes our QFE system and its implementation. Our experiments and results are given in sects.Experimental Designand Results. Finally, we discuss limitations of QFE, possible improvements to QFE (including generalizations that QFE makes possible but that competing approaches would not), and our general conclusions.
Related Work
A variety of research has been conducted that uses GP either for the creation of queries (da Silva and Thomas, , among many others). Because this literature is quite voluminous and varied we will comment specifically only on those systems most closely related to QFE.
Castro da Silva and Thomas () directly evolve queries as individuals with the goal of generating queries for inexperienced SQL users. In order to ensure that evolved queries are syntactically correct they implement numerous non-standard genetic operators to combine and mutate individuals. This approach requires significant re-design of any existing GP system and, we would argue, limits the systems generality. Interestingly, this system seems to be the only prior work in which queries are allowed to include joins across tables, leaving the joining attribute up to evolution.
Acar and Motro () frame their work as trying to provide an alternative equivalent query to a given query by creating the alternative using the results of the original as positive examples. Although their motivation is different from ours, the resulting system has many similarities. Their method assumes that the sets of positive and negative examples cover the entire database instead of a small subset of it. The user must provide the entire set of example tuples that are in the database, which is probably impossible without using an a priori query to fetch them. The given system evolves actual queries as individuals, but can only handle queries expressible as trees of relational algebra expressions.
Freitas describes a GP system that evolves programs that can be interpreted as SQL queries to be used in thedata mining tasks ofclassification and generalized rule induction (Freitas, ). Individuals are represented as trees that directly correspond to WHERE clauses of queries. Unlike QFE, this work allows for the evolution of non-binary classifiers via niches that correspond to classes of the goal attribute. This paper was pioneering insofar as it introduced the idea of evolving SQL queries but it presents no experiments or results, and it does not make clear how one can deal with practical issues such as the choosing of constants, the design of an appropriate fitness function, the alterations that must be made to standard genetic operators, etc. Because there are no results one cannot judge the system with respect to query accuracy, comprehensibility, conciseness, and time. Additionally, this approach is limited (unlike the developmental approach that we present below) to the production of queries over a single table with WHERE clauses that can be expressed as trees. Freitas has continued to produce a great deal of significant related work but not, so far as we are aware, additional work on the use of GP to evolve SQL queries.