Volume 20
Springer Topics in Signal Processing
Series Editors
Jacob Benesty
Montreal, Canada
Walter Kellermann
Erlangen, Germany
More information about this series at http://www.springer.com/series/8109 The aim of the Springer Topics in Signal Processing series is to publish very high quality theoretical works, new developments, and advances in the field of signal processing research. Important applications of signal processing are covered as well. Within the scope of the series are textbooks, monographs, and edited books.
Topics include but are not limited to:
* Audio & Acoustic Signal Processing
* Biomedical Signal & Image Processing
* Design & Implementation of Signal Processing
* Graph Theory & Signal Processing
* Industrial Signal Processing
* Machine Learning for Signal Processing
* Multimedia Signal Processing
* Quantum Signal Processing
* Remote Sensing & Signal Processing
* Sensor Array & Multichannel Signal Processing
* Signal Processing for Big Data
* Signal Processing for Communication & Networking
* Signal Processing for Cyber Security
* Signal Processing for Education* Signal Processing for Smart Systems
* Signal Processing Implementation
* Signal Processing Theory & Methods
* Spoken language processing
** Indexing: The books of this series are indexed in Scopus and zbMATH **
Jacob Benesty , Israel Cohen and Jingdong Chen
Array Beamforming with Linear Difference Equations
1st ed. 2021
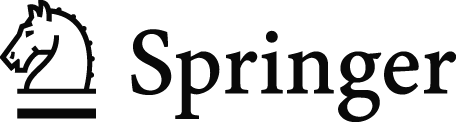
Logo of the publisher
Jacob Benesty
INRS-EMT, University of Quebec, Montreal, QC, Canada
Israel Cohen
Israel Institute of Technology, Haifa, Israel
Jingdong Chen
Northwestern Polytechnical University, Xian, China
ISSN 1866-2609 e-ISSN 1866-2617
Springer Topics in Signal Processing
ISBN 978-3-030-68272-9 e-ISBN 978-3-030-68273-6
https://doi.org/10.1007/978-3-030-68273-6
The Editor(s) (if applicable) and The Author(s), under exclusive license to Springer Nature Switzerland AG 2021
This work is subject to copyright. All rights are solely and exclusively licensed by the Publisher, whether the whole or part of the material is concerned, specifically the rights of translation, reprinting, reuse of illustrations, recitation, broadcasting, reproduction on microfilms or in any other physical way, and transmission or information storage and retrieval, electronic adaptation, computer software, or by similar or dissimilar methodology now known or hereafter developed.
The use of general descriptive names, registered names, trademarks, service marks, etc. in this publication does not imply, even in the absence of a specific statement, that such names are exempt from the relevant protective laws and regulations and therefore free for general use.
The publisher, the authors and the editors are safe to assume that the advice and information in this book are believed to be true and accurate at the date of publication. Neither the publisher nor the authors or the editors give a warranty, expressed or implied, with respect to the material contained herein or for any errors or omissions that may have been made. The publisher remains neutral with regard to jurisdictional claims in published maps and institutional affiliations.
This Springer imprint is published by the registered company Springer Nature Switzerland AG
The registered company address is: Gewerbestrasse 11, 6330 Cham, Switzerland
Abstract
Voice communication and human-machine speech interface systems have to face the problem of sound acquisition in complex acoustic environments, where noise, reverberation, and competing sources may coexist. To deal with this challenging issue, microphone arrays, which consist of multiple sensors arranged into a certain one-, two-, or three-dimensional topology, have to be used. The central component of an array system is signal processing, which operates on the signals observed by the array of sensors to restore a signal of interest while suppressing the unwanted signal components. Ideally, one would wish to simply improve the signal-to-noise ratio (SNR) without adding any distortion to either the signal of interest or the unwanted signals, thereby preserving the fidelity of both the signal and ambience. However, this seemingly easy objective turns out to be extremely difficult to achieve. With all the methods and techniques developed in the literature, signal enhancement is generally achieved by adding some degree of distortion to either the signal of interest or the ambience or even both at the same time. There is one exception: differential beamforming, whose root can be traced back to the principle of directional microphones invented in the 1940s; this principle was then introduced into the regime of microphone arrays in the 1990s to form differential microphone arrays (DMAs), which are designed to measure the spatial derivatives of the sound pressure field. Basically, a first-order DMA consists of two closely spaced omnidirectional pressure microphones, and its output is the subtraction of one sensors output from that of the other. The two omnidirectional microphones measure the sound pressure field, each from its own viewpoint. Since they are closely spaced, the difference between their outputs approximates very well the spatial derivative of the sound pressure field along the axis that connects the two sensors. So, this simple subtraction operation yields a differential beamformer that is responsive to the first-order differential of the acoustic pressure field. Similarly, a general Nth-order DMA can be formed by subtractively combining the outputs of two DMAs of order N1. This simple way of constructing microphone arrays has laid out the foundation for differential beamforming. The resulting beamformers feature a prominent property, that is, their beampatterns are frequency independent by theory, and have a great potential for use in high fidelity sound acquisition and enhancement as they do not add spectral distortion to the source signals regardless of the source incidence angle. A great deal of effort has been devoted to the study and design of differential beamformers over the last two decades. Many methods have been developed, most of which attempt to design the beamforming filter from the beampattern perspective, that is, the parameters of the beamformers are determined in such a way that the resulting beampattern matches well a target frequency-independent beampattern. Interestingly, few works have attempted to address the problem from the difference equations perspective, though this view is the foundation for differential beamforming. This book is intended to study the link between differential beamforming and differential equations, which is missed in the literature. This link enables to study the fundamental theory and methods of beamforming from a rather different perspective, leading to new insights into the problem and new methods to solve the problem. The book first presents a brief overview of the problems and methods for beamforming and some performance measures popularly used either to evaluate beamformers or to derive optimal beamformers. Then, first-order, second-order, and general high-order linear difference equations are discussed, based on which we show how to formulate the beamforming problem and derive different beamforming methods, including fixed and adaptive ones. Furthermore, we show how to apply the theory of difference equations to the general problem of speech enhancement and deduce a number of noise reduction filters, including the maximum SNR filter, the Wiener filter, the MVDR filter, etc. Also covered in the book are the difference equations and differential beamforming from the spectral graph perspective.