Volume 82
Studies in Big Data
Series Editor
Janusz Kacprzyk
Polish Academy of Sciences, Warsaw, Poland
The series "Studies in Big Data" (SBD) publishes new developments and advances in the various areas of Big Data- quickly and with a high quality. The intent is to cover the theory, research, development, and applications of Big Data, as embedded in the fields of engineering, computer science, physics, economics and life sciences. The books of the series refer to the analysis and understanding of large, complex, and/or distributed data sets generated from recent digital sources coming from sensors or other physical instruments as well as simulations, crowd sourcing, social networks or other internet transactions, such as emails or video click streams and other. The series contains monographs, lecture notes and edited volumes in Big Data spanning the areas of computational intelligence including neural networks, evolutionary computation, soft computing, fuzzy systems, as well as artificial intelligence, data mining, modern statistics and Operations research, as well as self-organizing systems. Of particular value to both the contributors and the readership are the short publication timeframe and the world-wide distribution, which enable both wide and rapid dissemination of research output.
The books of this series are reviewed in a single blind peer review process.
Indexed by zbMATH.
All books published in the series are submitted for consideration in Web of Science.
More information about this series at http://www.springer.com/series/11970
Editors
Pardeep Kumar and Amit Kumar Singh
Machine Learning for Intelligent Multimedia Analytics
Techniques and Applications
1st ed. 2021
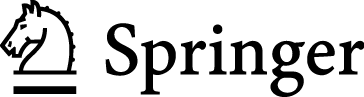
Logo of the publisher
Editors
Pardeep Kumar
Department of Computer Science and Engineering and Information Technology, Jaypee University of Information Technology, Solan, Himachal Pradesh, India
Amit Kumar Singh
Department of Computer Science and Engineering, National Institute of Technology, Patna, Bihar, India
ISSN 2197-6503 e-ISSN 2197-6511
Studies in Big Data
ISBN 978-981-15-9491-5 e-ISBN 978-981-15-9492-2
https://doi.org/10.1007/978-981-15-9492-2
Springer Nature Singapore Pte Ltd. 2021
This work is subject to copyright. All rights are reserved by the Publisher, whether the whole or part of the material is concerned, specifically the rights of translation, reprinting, reuse of illustrations, recitation, broadcasting, reproduction on microfilms or in any other physical way, and transmission or information storage and retrieval, electronic adaptation, computer software, or by similar or dissimilar methodology now known or hereafter developed.
The use of general descriptive names, registered names, trademarks, service marks, etc. in this publication does not imply, even in the absence of a specific statement, that such names are exempt from the relevant protective laws and regulations and therefore free for general use.
The publisher, the authors and the editors are safe to assume that the advice and information in this book are believed to be true and accurate at the date of publication. Neither the publisher nor the authors or the editors give a warranty, expressed or implied, with respect to the material contained herein or for any errors or omissions that may have been made. The publisher remains neutral with regard to jurisdictional claims in published maps and institutional affiliations.
This Springer imprint is published by the registered company Springer Nature Singapore Pte Ltd.
The registered company address is: 152 Beach Road, #21-01/04 Gateway East, Singapore 189721, Singapore
Preface
Recently, multimedia such as text, image, audio, video, graphics stands as one of the most demanding and exciting aspects of the information era. Surveys established that every second a lot of multimedia information are created, published and transmitted explosively, becoming an indispensable part of todays big data. Such large-scale multimedia data has disclosed new challenges and opportunities for intelligent multimedia analysis. It provides innovative opportunities to address several potential research problems, e.g., enabling comprehensive visual classification to fill the semantic gap by exploring large-scale data, offering a promising frontier for detailed multimedia understanding, as well as extract patterns and making effective decisions by analyzing the large collection of data. The processing of multimedia large-scale data has emerged as a most demanding area for the application of machine learning techniques. Therefore, the machine learning for intelligent multimedia analytics is becoming an emerging research area for multimedia research community.
Outline of the Book and Chapter Synopsis
In view of above, this book presents state-of-the-art intelligent techniques and approaches, design, development and innovative use of machine learning techniques for demanding applications of intelligent multimedia analytics. We have provided potential thoughts and methodologies that help senior undergraduate and graduate students, researchers, programmers and industry professionals in creating new knowledge for the future to develop intelligent multimedia analytics-based novel approach for multimedia applications. Further, key role and great importance of machine learning techniques as a mathematical tool is elaborated in the book. A brief and orderly introduction to the chapters is provided in the following. The book contains fifteen chapters.
Chapter presents a multi-modal technique that uses 2D and 3D ear images for secure access. A two-stage, coarse and fine alignment to fit the 3D ear image of the probe to the 3D ear image of the gallery. The probe and gallery image keypoints are compared using feature vectors, where very similar keypoints are used as coarse alignment correspondence points. Once the ear pairs are matched fairly closely, the entire data is finely aligned to compute the matching score. A detailed systematic analysis using a large ear database has been carried out to show the efficiency of the technique proposed.
Chapter presents efficient and low overhead detection of brain diseases using deep learning-based sparse MRI image classification for four different brain diseases like edema, necrosis, enhancing and non-enhancing tumor. The main contribution of the this book chapter lies in utilizing the sparsity of the set of images that reduces the training and inference time of the CNN.
Chapter introduces continual deep learning framework for medical media screening and archival. The primary section of the proposed piece of work helps us to automatically diagnose tuberculosis (TB) from chest X-rays in minimal execution time and memory footprint. Secondarily, medical media screening and analysis would drive the process of automated archival strategy, tagged to self-generated metadata. Gradual self-improvement of the AI engine narrowing down the fuzzy zone between confident positive and confident negative of diagnosis would be the key achievement of proposed continuous deep learning framework.