Thomas Barrau - Artificial Intelligence for Financial Markets: The Polymodel Approach
Here you can read online Thomas Barrau - Artificial Intelligence for Financial Markets: The Polymodel Approach full text of the book (entire story) in english for free. Download pdf and epub, get meaning, cover and reviews about this ebook. year: 2022, publisher: Springer, genre: Romance novel. Description of the work, (preface) as well as reviews are available. Best literature library LitArk.com created for fans of good reading and offers a wide selection of genres:
Romance novel
Science fiction
Adventure
Detective
Science
History
Home and family
Prose
Art
Politics
Computer
Non-fiction
Religion
Business
Children
Humor
Choose a favorite category and find really read worthwhile books. Enjoy immersion in the world of imagination, feel the emotions of the characters or learn something new for yourself, make an fascinating discovery.
- Book:Artificial Intelligence for Financial Markets: The Polymodel Approach
- Author:
- Publisher:Springer
- Genre:
- Year:2022
- Rating:3 / 5
- Favourites:Add to favourites
- Your mark:
- 60
- 1
- 2
- 3
- 4
- 5
Artificial Intelligence for Financial Markets: The Polymodel Approach: summary, description and annotation
We offer to read an annotation, description, summary or preface (depends on what the author of the book "Artificial Intelligence for Financial Markets: The Polymodel Approach" wrote himself). If you haven't found the necessary information about the book — write in the comments, we will try to find it.
Thomas Barrau: author's other books
Who wrote Artificial Intelligence for Financial Markets: The Polymodel Approach? Find out the surname, the name of the author of the book and a list of all author's works by series.
Artificial Intelligence for Financial Markets: The Polymodel Approach — read online for free the complete book (whole text) full work
Below is the text of the book, divided by pages. System saving the place of the last page read, allows you to conveniently read the book "Artificial Intelligence for Financial Markets: The Polymodel Approach" online for free, without having to search again every time where you left off. Put a bookmark, and you can go to the page where you finished reading at any time.
Font size:
Interval:
Bookmark:
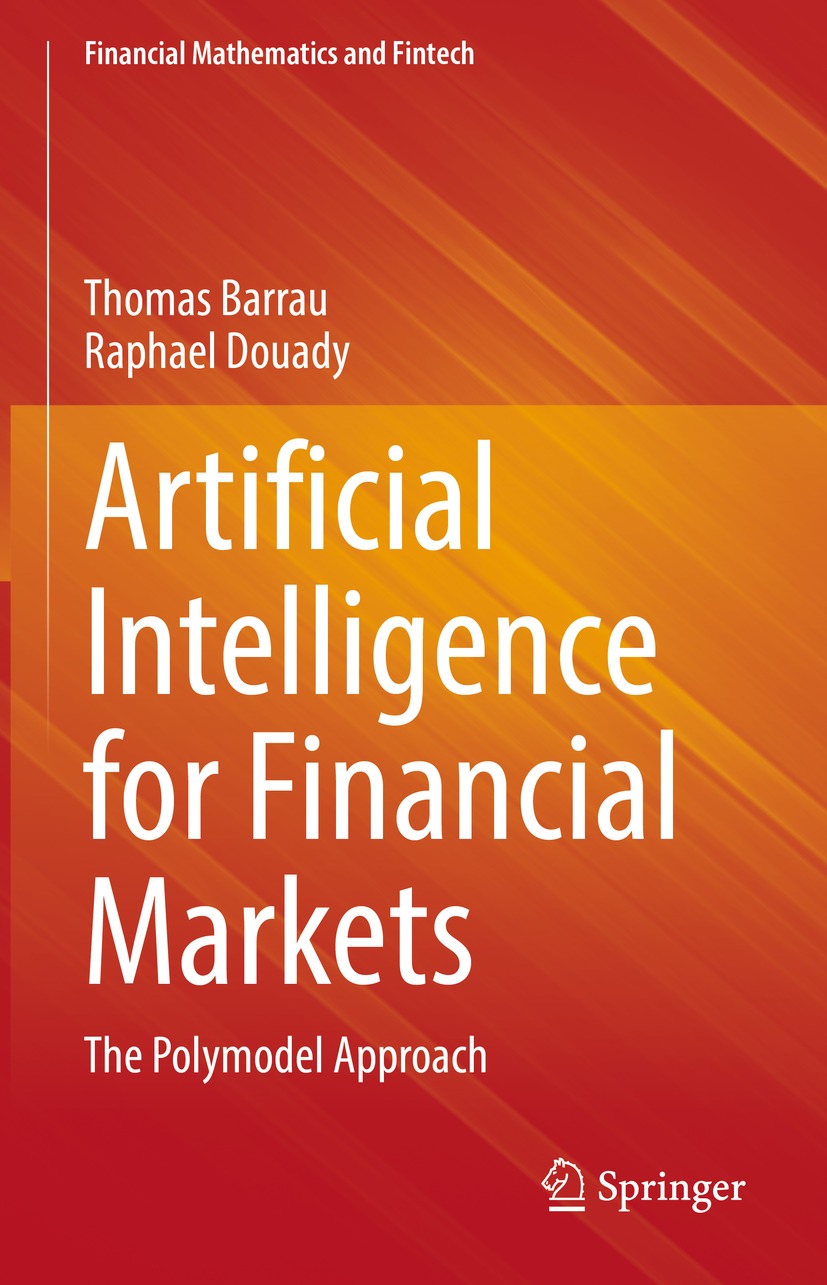
This series addresses the emerging advances in mathematical theory related to finance and application research from all the fintech perspectives. It is a series of monographs and contributed volumes focusing on the in-depth exploration of financial mathematics such as applied mathematics, statistics, optimization, and scientific computation, and fintech applications such as artificial intelligence, block chain, cloud computing, and big data. This series is featured by the comprehensive understanding and practical application of financial mathematics and fintech. This book series involves cutting-edge applications of financial mathematics and fintech in practical programs and companies.
The Financial Mathematics and Fintech book series promotes the exchange of emerging theory and technology of financial mathematics and fintech between academia and financial practitioner. It aims to provide a timely reflection of the state of art in mathematics and computer science facing to the application of finance. As a collection, this book series provides valuable resources to a wide audience in academia, the finance community, government employees related to finance and anyone else looking to expand their knowledge in financial mathematics and fintech.
The key words in this series include but are not limited to:
a) Financial mathematics
b) Fintech
c) Computer science
d) Artificial intelligence
e) Big data
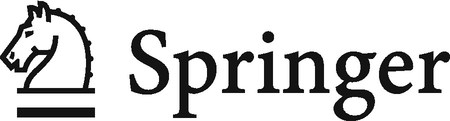
This Springer imprint is published by the registered company Springer Nature Switzerland AG
The registered company address is: Gewerbestrasse 11, 6330 Cham, Switzerland
This book exposes a manageable approach to exploit nonlinearity for the purpose of financial investment. It summarizes the research Raphael Douady and co-authors have conducted for nearly two decades now. This research is expanded on multiple aspects by a joint work conducted by Thomas Barrau.
In general, relationships between variables are nonlinear and noisy. When attempting to relate a target variable to several explanatory variables, modeling finely multi-dimensional linearity suffers from the curse of dimensionality without bringing blatant benefits. Polymodels simplify this approach by retaining unidimensional nonlinearities and proposing various aggregation methods according to the task at hand. For instance, in the case of prediction, each one-dimensional model produces a prediction. The most relevant ones are then selected, then possibly averaged in case of ambiguity, to yield the final prediction. In the case of defining a risk indicator, as downturns are usually associated with an increase of correlations, the distribution of goodness-of-fit metrics is analyzed. It can be seen as a form of ensemble learning.
The first task is to perform the one-dimensional nonlinear regressions. Several methods are presented, including non-parametric kernel regressions but the method of choice is linear regression on nonlinear functions (Hermite polynomials for their orthogonality properties) of the explanatory variable, further regularized by shrinkage to the linear component to mitigate overfitting.
Nonlinear transforms of initial factors can be used as new variables or features to feed a machine learning algorithm. Another use of extracted nonlinearities is to transform their convexity into an antifragility score (in the sense of N.N. Taleb). These scores can then be used as a factor to build long-short portfolios. The Polymodel approach can be implemented at the level of the market, industries, or individual stocks. With the help of Bayesian conditioning it can combine the information of hundreds of factors.
In summary, polymodels provide a very workable methodology to exploit nonlinearity for the purpose of investing, forecasting and risk management. Barrau and Douady describe the method and its application in this book filled with examples and backtests which will certainly inspire numerous finance practitioners.
Like many innovative ideas, polymodels emerged from a very stressful situation. Soon after we started the Riskdata venture, a new risk software aimed at the buy-side, the Twin Towers in New York were hit. This event would change the face of the world, and seriously put our nascent project at stake. There was no chance to sign a single contract for at least a year. While we were about to abandon the project and find a payroll job, a fund of hedge fund manager proposed the following challenge to us: given only audited monthly returns of the funds, what can we reliably say about their strategy, their risks, and how they fit in a portfolio, made of both liquid and alternative assets?
This challenge was way beyond the mere assessment of classical performance metrics, such as the Sharpe ratio, downside volatility, beta, FamaFrench factor analysis, etc. These measures, widely used by the industry, are known to provide relevant information from the past, but nothing truly reliable for the future behavior. We figured out that, if a software, however sophisticated, ignores the key points addressed in a conversation between an investor and a manager, it would miss the elephant in the room. So, we asked this manager to let us silently attend some of his meetings with the managers he was either invested in or planning to. To our surprise, the investor would spend only a few minutes talking about correlations and volatility, then the rest of the hour on specific moments that were relevant to the particular strategy: how did the manager weather such or such an event?; when and how did he flip his positions?; and other such questions. Our challenge was to put these questions into a software that was systematic enough to automatically address them. The idea was
Font size:
Interval:
Bookmark:
Similar books «Artificial Intelligence for Financial Markets: The Polymodel Approach»
Look at similar books to Artificial Intelligence for Financial Markets: The Polymodel Approach. We have selected literature similar in name and meaning in the hope of providing readers with more options to find new, interesting, not yet read works.
Discussion, reviews of the book Artificial Intelligence for Financial Markets: The Polymodel Approach and just readers' own opinions. Leave your comments, write what you think about the work, its meaning or the main characters. Specify what exactly you liked and what you didn't like, and why you think so.