Ervin Varga - Synthesizing Actionable Insights from Data
Here you can read online Ervin Varga - Synthesizing Actionable Insights from Data full text of the book (entire story) in english for free. Download pdf and epub, get meaning, cover and reviews about this ebook. year: 2019, publisher: Apress, genre: Computer. Description of the work, (preface) as well as reviews are available. Best literature library LitArk.com created for fans of good reading and offers a wide selection of genres:
Romance novel
Science fiction
Adventure
Detective
Science
History
Home and family
Prose
Art
Politics
Computer
Non-fiction
Religion
Business
Children
Humor
Choose a favorite category and find really read worthwhile books. Enjoy immersion in the world of imagination, feel the emotions of the characters or learn something new for yourself, make an fascinating discovery.
- Book:Synthesizing Actionable Insights from Data
- Author:
- Publisher:Apress
- Genre:
- Year:2019
- Rating:4 / 5
- Favourites:Add to favourites
- Your mark:
- 80
- 1
- 2
- 3
- 4
- 5
Synthesizing Actionable Insights from Data: summary, description and annotation
We offer to read an annotation, description, summary or preface (depends on what the author of the book "Synthesizing Actionable Insights from Data" wrote himself). If you haven't found the necessary information about the book — write in the comments, we will try to find it.
Synthesizing Actionable Insights from Data — read online for free the complete book (whole text) full work
Below is the text of the book, divided by pages. System saving the place of the last page read, allows you to conveniently read the book "Synthesizing Actionable Insights from Data" online for free, without having to search again every time where you left off. Put a bookmark, and you can go to the page where you finished reading at any time.
Font size:
Interval:
Bookmark:
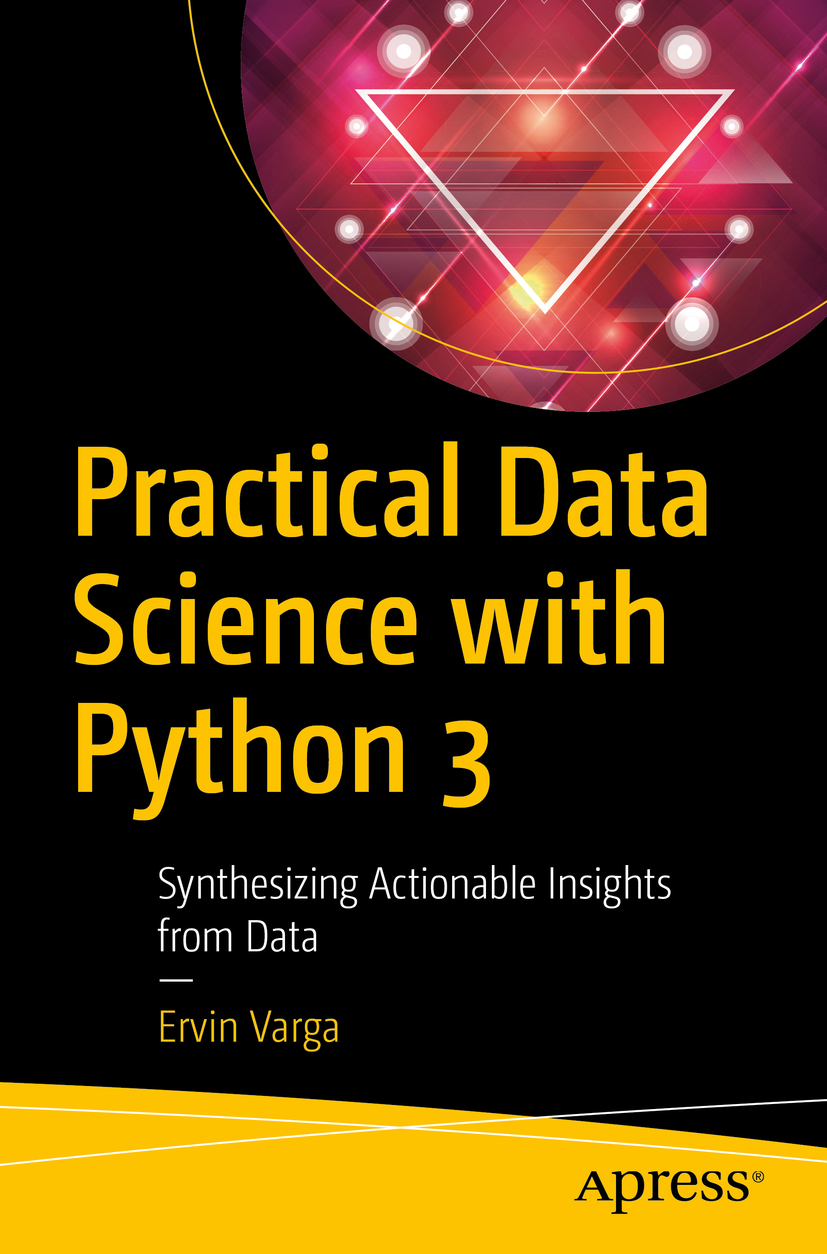
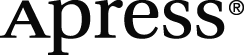
Any source code or other supplementary material referenced by the author in this book is available to readers on GitHub via the books product page, located at www.apress.com/978-1-4842-4858-4 . For more detailed information, please visit http://www.apress.com/source-code .
Like traveling, writing a book is more enjoyable when accompanied by your family. I am thankful to my wife, Zorica, and sons, Andrej and Stefan, for all their great support.
This book amalgamates data science and software engineering in a pragmatic manner. It guides the reader through topics from these worlds and exemplifies concepts through software. As a reader, you will gain insight into areas rarely covered in textbooks, since they are hard to explain and illustrate. You will see the Cynefin framework in action via examples that give an overarching context and systematic approach for your data science endeavors.
The book also introduces you to the most useful Python 3 data science frameworks and tools: Numpy, Pandas, scikit-learn, matplotlib, Seaborn, Dask, Apache Spark, PyTorch, and other auxiliary frameworks. All examples are self-contained and allow you to reproduce every piece of content from the book, including graphs. The exercises at the end of each chapter advise you how to further deepen your knowledge.
Finally, the book explains, again using lots of examples, all phases of a data science life cycle model: from project initiation to data exploration and retrospection. The aim is to equip you with necessary comprehension pertaining to major areas of data science so that you may see the forest for the trees .
I would like to thank Apress for giving me an opportunity and full support for writing this book about data science. Comments and help from James Markham, Aditee Mirashi, and Celestin Suresh John were invaluable.
I am also grateful for excellent remarks from Jojo John Moolayil, who was the technical reviewer on this book.
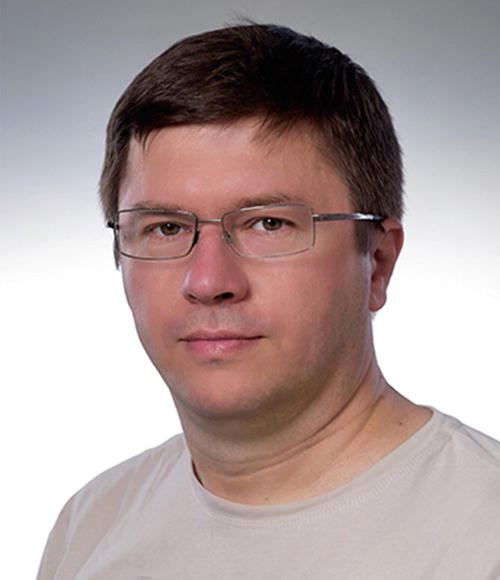
is a Senior Member of IEEE and Professional Member of ACM. He is an IEEE Software Engineering Certified Instructor. Ervin is an owner of the software consulting company Expro I.T. Consulting, Serbia. He has an MSc in computer science, and a PhD in electrical engineering (his thesis was an application of software engineering and computer science in the domain of electrical power systems). Ervin is also a technical advisor of the open-source project Mainflux.
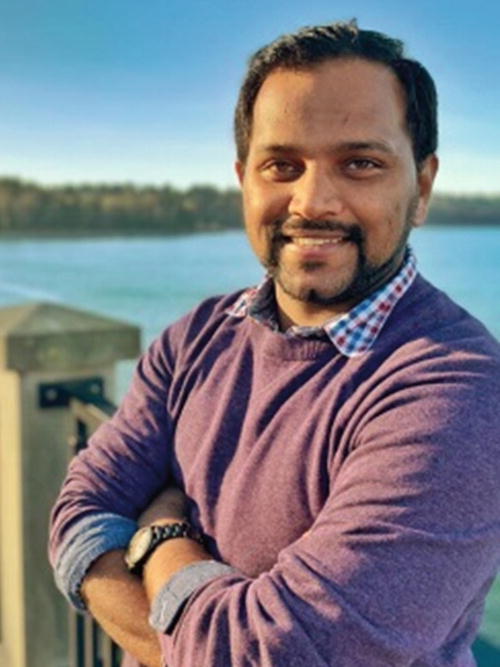
is an artificial intelligence professional and published author of three books on machine learning, deep learning, and IoT. He is currently working with Amazon Web Services as a Research Scientist AI in AWSs office in Vancouver, BC.
Jojo was born and raised in Pune, India, and graduated from the University of Pune with a major in Information Technology Engineering. His passion for problem-solving and data-driven decision-making led him to start a career with Mu Sigma Inc., the worlds largest pure-play analytics provider. There, he was responsible for developing machine learning and decision science solutions for large, complex problems for healthcare and telecom giants. He later worked with Flutura (an IoT analytics startup) and General Electric with a focus on industrial AI, in Bangalore, India.
In his current role with AWS, he works on researching and developing large-scale AI solutions for combating fraud and enriching the customers payment experience in the cloud. He is also actively involved as a tech reviewer and AI consultant with leading publishers and has reviewed over a dozen books on machine learning, deep learning, and business analytics.
You can reach out to Jojo at https://www.jojomoolayil.com/ , https://www.linkedin.com/in/jojo62000 , and https://twitter.com/jojo62000 .
Let me start by making an analogy between software engineering and data science. Software engineering may be summarized as the application of engineering principles and methods to the development of software. The aim is to produce a dependable software product. In a similar vein, data science may be described as the application of scientific principles and methods to data collection, analysis, and reporting. The goal is to synthesize reliable and actionable insights from data (sometimes referred as data product). To continue with our analogy, the systems/software development life cycle (SDLC) prescribes the major phases of a software development process: project initiation, requirements engineering, design, construction, testing, deployment, and maintenance. The data science process also encompasses multiple phases: project initiation, data acquisition, data preparation, data analysis, reporting, and execution of actions (another phase is data exploration, which is more of an all-embracing activity than a stand-alone phase). As in software development, these phases are quite interwoven, and the process is inherently iterative and incremental. An overarching activity that is indispensable in both software engineering and data science (and any other iterative and incremental endeavor) is
Font size:
Interval:
Bookmark:
Similar books «Synthesizing Actionable Insights from Data»
Look at similar books to Synthesizing Actionable Insights from Data. We have selected literature similar in name and meaning in the hope of providing readers with more options to find new, interesting, not yet read works.
Discussion, reviews of the book Synthesizing Actionable Insights from Data and just readers' own opinions. Leave your comments, write what you think about the work, its meaning or the main characters. Specify what exactly you liked and what you didn't like, and why you think so.