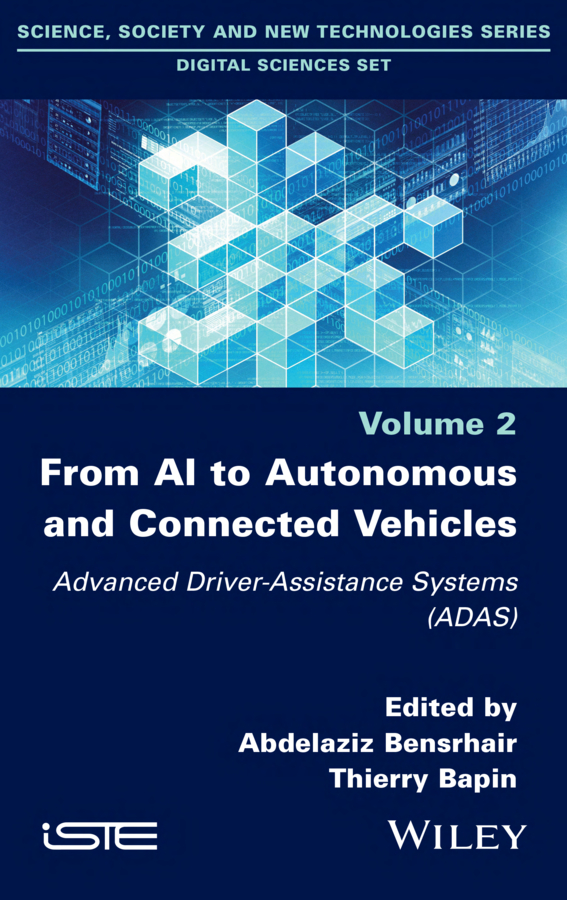
Digital Sciences Set
coordinated by
Abdelkhalak El Hami
Volume 2
From AI to Autonomous and Connected Vehicles
Advanced Driver-Assistance Systems (ADAS)
Edited by
Abdelaziz Bensrhair
Thierry Bapin

First published 2021 in Great Britain and the United States by ISTE Ltd and John Wiley & Sons, Inc.
Apart from any fair dealing for the purposes of research or private study, or criticism or review, as permitted under the Copyright, Designs and Patents Act 1988, this publication may only be reproduced, stored or transmitted, in any form or by any means, with the prior permission in writing of the publishers, or in the case of reprographic reproduction in accordance with the terms and licenses issued by the CLA. Enquiries concerning reproduction outside these terms should be sent to the publishers at the undermentioned address:
ISTE Ltd
27-37 St Georges Road
London SW19 4EU
UK
www.iste.co.uk
John Wiley & Sons, Inc.
111 River Street
Hoboken, NJ 07030
USA
www.wiley.com
ISTE Ltd 2021
The rights of Abdelaziz Bensrhair and Thierry Bapin to be identified as the authors of this work have been asserted by them in accordance with the Copyright, Designs and Patents Act 1988.
Library of Congress Control Number: 2021938230
British Library Cataloguing-in-Publication Data
A CIP record for this book is available from the British Library
ISBN 978-1-78630-727-9
Foreword 1
This book is the result of many collaborations between the engineering school, INSA Rouen Normandie, and the French cluster for automotive & mobility industry, NextMove.
The subject of the book concerns the field of driving automation (ADAS, advanced driver-assistance systems), in conjunction with the connected and autonomous vehicle (CAV) educational chair.
Created in June 2006 and located in the Normandy and le-de-France regions (where 70% of French automotive R&D is carried out), NextMove embodies, animates and promotes the Mobility Valley, an area of European excellence where solutions are invented, developed, tested and industrialized to meet the challenges of mobility today and tomorrow. Convinced that the future of mobility players in France depends on their ability to be competitive through innovation, NextMove weaves and animates a dynamic network between major manufacturers, SMEs, start-ups, higher education, research institutions and local authorities. NextMove brings together more than 600 member institutions and supports more than 500 labeled projects (including more than 250 funded projects). NextMove is the largest French network of scientific and technical excellence, bringing together more than 600 members from the French mobility and automotive industry.
It is within this ecosystem which encourages innovation that the idea was born to create an educational chair of excellence devoted to the study of the CAV, bringing together the SMEs of Groupement ADAS and INSA Rouen Normandie. At the origin of this initiative, there was a convergence of views between Grard Yahiaoui SME VP of NextMove and University Professor Abdelaziz Benshrair, who agreed on the need to bring together high-tech SMEs and students of major engineering schools. Traditionally reserved for large companies, chairs previously seemed inaccessible to start-ups and SMEs due to the amount of funding. Thanks to the critical size of the Groupement ADAS and the common will of all the actors, this CAV chair was able to take shape.
Created at the initiative of NextMove, Groupement ADAS brings together several innovative SMEs in the field of driving assistance systems and autonomous vehicles. Its mission is to bring electronic and digital innovation to vehicles, to meet the challenges of road safety and driving comfort. Groupement ADAS provides embedded products, multidisciplinary expertise and interoperable open technologies. To continue to drive innovation, the recruitment of future talents is a key issue.
Indeed, the challenges to be met in the field of automated, autonomous and assisted mobility increasingly require the training of young engineers in new technologies. Innovation is not only the prerogative of major groups. Thanks to the creation of the Connected & Autonomous Vehicle educational chair, students will be able to choose this specialization during their fifth year studying computer science. For this, high-tech start-ups and SMEs will provide part of the 42 hours of courses proposed. Embedded perception, the modeling of the drivers behavior, the automation of driving and deep learning are all topics covered in this specialization. The possibility of being able to exchange daily with leaders of high-tech SMEs in the mobility sector will allow them to become aware of the formidable opportunities for expressing their talents, by tackling concrete cases during their internship.
Thierry BAPIN
NextMove, the French competitiveness cluster
for the automotive & mobility industry
May 2021
Foreword 2
AI and ADAS, How Can Mobility be Improved?
The mobility context
Numerous statistical studies have been carried out over the last decade in France, Europe and around the world in order to quantify the number of road accidents and what causes them. At the European level (European Commission 2019), the problem is significant, with a total 1 million road accidents, 1.4 million injuries and 25,600 deaths per year. At a global scale, road accidents cause 1.35 million deaths and 20 to 50 million serious injuries or lead to a disability every year (World Health Organization 2019). In the case of France, there has been an average of 3,400 deaths per year for several years. But if we take into account the amount of people injured, the number rises to 70,000 casualties and injured persons. This represents a significant cost for society.
In addition, it clearly appears that the majority of accidents involve a human factor, including the driver (93%). These studies also show the predominance of a set of factors related to the drivers abilities and other factors deteriorate their ability to drive. These may be related to perception, interpretation, assessment, decision-making problems and, ultimately, problems related to their actions. More concretely, the driver may lack visibility, or due to the complexity of road situations have limited and/or superficial information, leading to a poor understanding or misinterpretation of the scene. In addition, there are also problems triggered by the deliberate violation of The Highway Code, alcoholism and drug abuse, lack of driving experience, and also fatigue, hypovigilance, monotony, or they may even just be distracted.
It is therefore essential to be able to help the driver in order to reduce the number of road accidents, as well as to minimize risk in road situations. This reduction can be applied by providing the driver with both informative (alerts, instructions, expertise) and active (automation, delegation and shared driving) assistance. These aids are intended to help, as much as possible, the drivers limitations regarding perception, decision-making, and their actions, without forgetting compensation for the drivers potential failures.
Perception and AI, endowing ADAS with sight and intelligence
These driving aids necessarily go through an essential and critical stage of perception. This type of perception must respond to a large number of constraints in order to guarantee a high level of performance, reliability and robustness. It must be dynamic and adaptive in order to be able to take into account the data coming from the embedded sensors, depending on their relevance, quality and availability. After being processed, this information enables the construction of cooperative local and global dynamic perception maps containing an estimate of the attributes of the key components involved in the road scene. In the representation of the road environment, these key components are made up of obstacles, the road, the ego-vehicle, the environment and finally, the driver. Having a knowledge of the state of all these key components makes it possible to have sufficiently comprehensive perception at our disposal, usable by a large number of embedded security applications in the short, medium and long term. However, active applications involved in the automation of the driving process require a truly high quality of service, ensuring the optimal reliability, confidence, accuracy and robustness of the attributes of road scene key components. For this, techniques based on the use of Artificial Intelligence (machine learning, deep learning, graph theory, expert systems, fuzzy logic, etc.) are being developed and applied for processing sensor data and enabling the production of detection, filtering, data restoration, tracking, identification and recognition functions. This processing is either applied per sensor or per sensor set (multi-sensor fusion). However, perception is often limited by the performance of embedded sensors and by the impact of adverse and degraded environmental conditions on the technologies used by the sensors. This is critical because a faulty or degraded sensor can jeopardize the proper operating of an active application like the ones used for automated driving systems. In fact, at levels 3 and 4 of the automation driving task, the driver is still present and at any time they can receive a request to intervene and to take over the driving task, when the system is no longer able to operate correctly. Unfortunately, it takes longer than 10 seconds (Merat 2014) for this transition (from virtual co-pilot mode to human pilot mode) to be achieved. This amount of time is necessary for an inattentive driver to perceive the current environment, to analyze and understand it, to make the best possible decision, and to act effectively on the vehicles actuators (pedals, steering wheel, and potentially, the gearbox). Thus, to be warned of a disengagement from the automated piloting system, it is necessary to have information that is sufficiently distant in terms of space and time so as to make it possible to analyze, interpret and predict the future situation, and estimate its level of risk. The most effective way to extend this perception is to use the knowledge available coming from the other road users. For this, it is necessary to use means of communication (transmitters and receivers embedded in the vehicles or present on the infrastructure (road side)).
Next page