Cause and Correlation in Biology
A User's Guide to Path Analysis, Structural Equations and Causal Inference with R
Second Edition
Many problems in biology require an understanding of the relationships among variables in a multivariate causal context. Exploring such causeeffect relationships through a series of statistical methods, this book explains how to test causal hypotheses when randomised experiments cannot be performed.
This completely revised and updated edition features detailed explanations for carrying out statistical methods using the popular, and freely available, R statistical language. Sections on d-sep tests, latent constructs that are common in biology, missing values, phylogenetic constraints and multilevel models are also an important feature of this new edition.
Written for biologists and using a minimum of statistical jargon, the concept of testing multivariate causal hypotheses using structural equations and path analysis is demystified. Assuming only a basic understanding of statistical analysis, this new edition is a valuable resource for students and practising biologists alike.
Bill Shipley is a Professor in the Department of Biology at Universit de Sherbrooke, Canada. His research interests centre upon plant ecophysiology, functional and community ecology and statistical modelling. He is the author of From Plant Traits to Vegetation Structure: Chance and Selection in the Assembly of Ecological Communities , published by Cambridge University Press.
Cause and Correlation in Biology
A User's Guide to Path Analysis, Structural Equations and Causal Inference with R
Second Edition
Bill Shipley
Universit de Sherbrooke, Canada
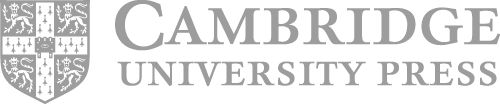
University Printing House, Cambridge CB2 8BS, United Kingdom
Cambridge University Press is part of the University of Cambridge.
It furthers the University's mission by disseminating knowledge in the pursuit of education, learning and research at the highest international levels of excellence.
www.cambridge.org
Information on this title: www.cambridge.org/9781107442597
Cambridge University Press 2016
This publication is in copyright. Subject to statutory exception and to the provisions of relevant collective licensing agreements, no reproduction of any part may take place without the written permission of Cambridge University Press.
First published 2000
Second edition 2016
Printed in the United Kingdom by Clays, St Ives plc
A catalogue record for this publication is available from the British Library
ISBN 978-1-107-44259-7 Paperback
Cambridge University Press has no responsibility for the persistence or accuracy of URLs for external or third-party internet websites referred to in this publication, and does not guarantee that any content on such websites is, or will remain, accurate or appropriate.
ma petite Rhinanthe toujours aussi belle, David et lyse.
Contents
Preface
This book describes a series of statistical methods for testing causal hypotheses using observational data but it is not a statistics book. It describes a series of algorithms, derived from research in artificial intelligence (AI), that can discover causal relationships from observational data but it is not a book about artificial intelligence. It describes the logical and philosophical relationships between causality and probability distributions but it is certainly not a book about the philosophy of statistics. Rather, it is a user's guide , written for biologists, whose purpose is to allow the practising biologist to make use of these important new developments when causal questions cannot be answered with randomised experiments.
I have written the book assuming that you have no previous training in these methods. If you have taken an introductory statistics course even if it was longer ago than you want to acknowledge and have managed to hold on to some of the basic notions of sampling and hypothesis testing using statistics then you should be able to understand the material in this book. I recommend that you read each chapter through in its entirety even if you do not feel that you have mastered all the notions. This will at least give you a general feeling for the goals and vocabulary of each chapter. You can then go back and pay closer attention to the details.
The book is addressed to biologists, mostly because I am a practising biologist myself, but I hope that it will also be of interest to statisticians, scientists in other fields and even philosophers of science. I have not written the book as a textbook simply because the discipline to which the material in this book naturally belongs does not yet exist. Whatever the name eventually given to this new discipline, I firmly believe that it will exist, and be generally recognised as a distinct discipline, in the future. The questions that this new discipline addresses, and the elegance of its results, are too important for this not to be the case. Nonetheless, the chapters follow a logical progression that would be well suited to an upper-level undergraduate, or graduate, course. I have used the manuscript of this book for such a purpose, and every one of my students is still alive.
It is a pleasure and an honour to acknowledge the many people who have contributed to this project. First, Jim and Marg Shipley started everything. Robert van Hulst supplied much of the initial impulse through our conversations about science and causality while I was still an undergraduate. He has also read every one of the manuscript chapters and suggested many useful changes. Paul Keddy kept my interest burning during my PhD studies and also commented on the first two chapters. As usual, his comments went to the heart of the matter.
The late Robert Peters had a large impact on my thoughts about causality and even convinced me, for a number of years, that ecologists are best to give up on the concept not because he viewed the notion of causality as meaningless (he never believed this, despite his empiricist reputation) but because it was simply too slippery a notion to demonstrate without randomised experiments. His constant prodding must have caused me to stop while wandering through the library one day when, almost subconsciously, I saw a book with the following provocative title: Discovering Causal Structure: Artificial Intelligence, Philosophy of Science, and Statistical Modeling (Glymour et al. 1987). That book was my introduction to a more sophisticated understanding of causality. Rob Peters was much too young when he passed away, and I am sorry that he never got to read the book that you are about to begin. I am not sure that he would have approved of everything in it but I know that he would have appreciated the effort.
Martin Lechowicz introduced me to the notion of path analysis. I must also acknowledge my graduate students, Margaret McKenna, Driss Meziane, Jarceline Almeida-Cortez, Luc St-Pierre and Muhaymina Sari, as well as the many members of the SEMNET Internet discussion group.
Finally, I want to thank Judea Pearl for kindly responding to my many e-mails about d-separation and basis sets and to Clark Glymour, Richard Scheines and Peter Spirtes of Carnegie Mellon University for their generosity in extending an invitation to visit with them and for patiently answering my many questions about their discovery algorithms. Clark Glymour read and commented on some of the manuscript chapters.
I hope that you find this book to be useful, interesting and readable. I welcome your comments and feedback especially if you don't agree with me.
Next page