Kenneth J. Berry , Kenneth L. Kvamme , Janis E. Johnston and Paul W. Mielke, Jr.
Permutation Statistical Methods with R
1st ed. 2021
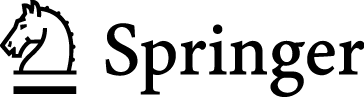
Logo of the publisher
Kenneth J. Berry
Department of Sociology, Colorado State University, Fort Collins, CO, USA
Kenneth L. Kvamme
Department of Anthropology, University of Arkansas, Fayetteville, AR, USA
Janis E. Johnston
Alexandria, VA, USA
Paul W. Mielke, Jr.
Deceased, Fort Collins, CO, USA
ISBN 978-3-030-74360-4 e-ISBN 978-3-030-74361-1
https://doi.org/10.1007/978-3-030-74361-1
The Editor(s) (if applicable) and The Author(s), under exclusive license to Springer Nature Switzerland AG 2021
This work is subject to copyright. All rights are solely and exclusively licensed by the Publisher, whether the whole or part of the material is concerned, specifically the rights of translation, reprinting, reuse of illustrations, recitation, broadcasting, reproduction on microfilms or in any other physical way, and transmission or information storage and retrieval, electronic adaptation, computer software, or by similar or dissimilar methodology now known or hereafter developed.
The use of general descriptive names, registered names, trademarks, service marks, etc. in this publication does not imply, even in the absence of a specific statement, that such names are exempt from the relevant protective laws and regulations and therefore free for general use.
The publisher, the authors and the editors are safe to assume that the advice and information in this book are believed to be true and accurate at the date of publication. Neither the publisher nor the authors or the editors give a warranty, expressed or implied, with respect to the material contained herein or for any errors or omissions that may have been made. The publisher remains neutral with regard to jurisdictional claims in published maps and institutional affiliations.
This Springer imprint is published by the registered company Springer Nature Switzerland AG
The registered company address is: Gewerbestrasse 11, 6330 Cham, Switzerland
Dr. Paul W. Mielke, Jr., Professor Emeritus of Statistics at Colorado State University and Fellow of the American Statistical Association, passed away on 20 April 2019.
For our families: Nancy T. Berry, Ellen E. Berry, and Laura B. Berry; JoAnn Kvamme; Kathie Lowry, Marlin Lowry, Brian Lowry, and Brent and Kappi Lowry; and Roberta R. Mielke, William W. Mielke, Emily (Mielke) Spear, and Lynn (Mielke) Basila.
Preface
Permutation Statistical Methods with R presents exact and Monte Carlo permutation statistical methods for generating probability values and measures of effect size for a variety of tests of differences, measures of correlation and association, and measures of goodness of fit. Throughout the book the emphasis is on permutation statistical methods, although the results of permutation analyses are always compared and contrasted with the results of conventional statistical analyses, with which the reader is assumed to be familiar. R Scripts are provided for each conventional test statistic and associated permutation test statistics throughout the book.
Included in tests of differences are one-sample tests, tests of differences for two independent samples, tests of differences for two matched samples, tests of differences for multiple independent samples, and tests of differences for multiple matched samples. Included in measures of correlation and association are simple linear correlation and regression, measures of effect size based on Pearsons chi-squared test statistic, and several other measures of effect size designed for the analysis of contingency tables. The arrangement of the book follows the structure of a typical introductory textbook in statistics: introduction, central tendency and variability, one-sample tests, tests for two independent samples, tests for two matched samples, completely-randomized analysis of variance designs, randomized-blocks analysis of variance designs, simple linear regression and correlation, and the analysis of goodness of fit and contingency.
Chapter is to familiarize the reader with the structure and content of the book and provide a brief introduction to the various permutation tests and measures presented in the following chapters.
Chapter provides an introduction to the R programming language and to RStudio. Although the book is primarily a book on permutation statistical methods and not a book on R per se, the fundamentals of R, as they pertain to permutation methods, are important to present and explain. While the R programming language provides intrinsic (built-in) functions for most conventional statistical tests and measures, the R scripts for permutation statistical tests and measures are not available as intrinsic functions in R and are, as far as the authors know, unique to this book.
Chapter provides an introduction to two models of statistical inference: the population model and the permutation model. Most introductory textbooks in statistics and statistical methods present only the NeymanPearson population model of statistical inference. The NeymanPearson population model of statistical inference is named for Jerzy Neyman (18941981) and Egon Pearson (18951980) and was designed to make inferences about population parameters and provide approximate probability values under the appropriate null hypotheses. The NeymanPearson model is characterized by the assumptions of random sampling, normally-distributed population(s), and homogeneity of variances, where appropriate.
While the NeymanPearson population model will be familiar to most readers and needs little or no introduction, the FisherPitman permutation model of statistical inference is less likely to be familiar to many readers. The FisherPitman permutation model of statistical inference is named for R. A. Fisher (18901962) and E. J. G. Pitman (18971993). In contrast to conventional statistical tests based on the NeymanPearson population model, tests based on the FisherPitman permutation model are distribution-free, entirely data-dependent, appropriate for nonrandom samples, provide exact probability values, and are ideal for small data sets. On the other hand, permutation statistical methods can be computationally intensive, often requiring many millions of calculations. Thus, for the FisherPitman permutation model, exact and Monte Carlo permutation methods are described and compared. Under the NeymanPearson population model, squared Euclidean scaling functions are mandated, while under the FisherPitman permutation model, ordinary Euclidean scaling functions are shown to provide robust alternatives to conventional squared Euclidean scaling functions.
Chapter presents six interactive R scripts for calculating the various measures of central tendency and variability under the NeymanPearson population model and the FisherPitman permutation model.
Chapter presents an introduction to the permutation analysis of one-sample tests. In general, one-sample tests attempt to invalidate a hypothesized value of a population parameter, such as a population mean. Under the NeymanPearson population model, Students conventional one-sample 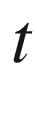